A) The Proceedings will be published in Digital Form.
B) Extended versions of the accepted Papers will be evaluated for possible publication in the following Journals
indexed in many reputable indexes, like Web of Science (Database: Emerging Sources Citation Index), SCOPUS, EI Compendex, IET, Google Scholar.
For the indexing of a particular journal visit its web page.
International Journal on Advanced Science, Engineering and Information Technology
http://ijaseit.insightsociety.org/
ARPN Journal of Engineering and Applied Sciences
http://www.arpnjournals.com/jeas/
International Journal of Applied Mathematics and Computer Science
https://www.amcs.uz.zgora.pl/
International Journal of Computers and Applications (Taylor and Francis)
http://www.tandfonline.com/toc/tjca20/current
Journal of Electrical Systems (JES)
http://journal.esrgroups.org/jes/
Journal of Theoretical and Applied Information Technology
http://www.jatit.org
Advances in Electrical and Electronic Engineering
http://advances.utc.sk/index.php/AEEE/index
TELKOMNIKA: Telecommunication, Computing, Electronics and Control
http://telkomnika.ee.uad.ac.id/
International Journal of Modern Manufacturing Technologies
http://www.ijmmt.ro/subscriptionsandexchanges.php
Indonesian Journal of Electrical Engineering and Computer Science (IJEECS)
http://www.iaescore.com/journals/index.php/IJEECS/index
Bulletin of Electrical Engineering and Informatics (BEEI)
http://journal.portalgaruda.org/index.php/EEI/index
Proceedings of the Institute of Mathematics and Mechanics
http://proc.imm.az/
Journal of Fundamental and Applied Sciences (JFAS)
http://www.jfas.info/index.php/jfas
Journal of Electrical Systems (JES)
http://journal.esrgroups.org/jes/
International Journal of Engineering and Technology
https://www.sciencepubco.com/index.php/ijet/index
International Journal of Power and Energy Systems
http://www.actapress.com/Content_of_Journal.aspx?JournalID=233
International Journal of Robotics and Automation
http://www.actapress.com/Content_of_Journal.aspx?JournalID=237
Reports of Biochemistry and Molecular Biology
http://rbmb.net/
Remote Sensing in Ecology and Conservation
https://zslpublications.onlinelibrary.wiley.com/journal/20563485
Quarterly Journal of Finance
https://www.worldscientific.com/worldscinet/qjf
International Journal of Electrical and Computer Engineering (IJECE)
http://iaesjournal.com/online/index.php/IJECE
International Journal of Power Electronics and Drive Systems
http://iaesjournal.com/online/index.php/IJPEDS
or in other similar journals indexed in:
Web of Science (Database: Emerging Sources Citation Index), SCOPUS, EI Compendex, IET, Google Scholar.
* Contact us by email:
support@inase.org Registration fees
Publication Ethics and Malpractice Statement
Deadlines
(Previous Conference: PMAMCM 2017, Agia Pelagia Beach, Crete, Greece, July 14-17, 2017)
PLENARY SPEAKERS:
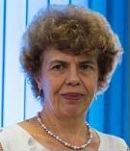
Prof. Dana Simian, University "Lucian Blaga" of Sibiu, Faculty of Sciences, Research Center on Informatics and Information Technology, ROMANIA, e-mail: dana.simian@ulbsibiu.ro
Title: "Optimization of Multiple Kernels in Support Vector Machines for Classification and Regression (SVMs and SVR)"
Abstract: The aim of this talk is to present a general approach for building optimal multiple kernels and for automatic optimization of the parameters in classification and regression based on support vectors, ie in Support Vector Machines (SVMs) and Support Vector Regression (SVR). SVMs are supervised learning methods introduced by Vapnik. Initialy SVMs were design for solving binary classification for linear separable data. SVMs construct, based on a training set of data, a linear classifyer with maximum margin. Training examples that are closest to this maximal separating hyperplane are named support vectors and they represents the only examples (vectors) which contribute to the expression of the classification hyperplane. SVMs were adapted for multi-class problems. For solving non-linear classification problems the data are mapped in a higher dimensional space where they become linearly separable. Using the "kernel trick" it is not necessary effectively to know this mapping. A kernel function is used for expressing the inner products involved in the equation of the separating hyperplane. SVR is a version of a SVM for regression. Both in SVM and SVR the choice of the kernel is extremely important for the prediction performances. Standard SVMs use one single kernel and the prediction supposes the choice of the kernel parameters. Usually the choice of the kernel is made in an empirical way. The real problems require more complex kernels. We propose and present an approach for building optimal multiple SVM and SVR kernels and automatic select optimal model parameters. We formalize our method and provide real applications and the conclusions of a sensitivity study.
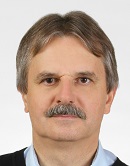
Prof. Janusz Brzdek, Department of Mathematics, Pedagogical University of Cracow, POLAND, e-mail: jbrzdek@up.krakow.pl
Title: "Approximate Solutions of Equations and Ulam Stability"
Abstract: The following natural question arises in many areas of scientific investigations: what errors we commit replacing the exact solutions to some equations by functions that satisfy those equations only approximately (or vice versa). Some efficient tools to evaluate those errors can be found in the theory of Ulam stability. The issue of Ulam stability of an equation can be very roughly expressed in the following way: when must a function satisfying an equation approximately (in some sense) be close (in a given way) to an exact solution to the equation? The problem of such stability was formulated for the first time by Ulam in 1940 for homomorphisms of metric groups; a solution to it was published a year later by Hyers for Banach spaces. Again in 1960, Ulam asked the following general question: when is it true that a solution of an equation differing slightly from a given one, must of necessity be close to a solution of the given equation? We discuss several issues connected to those questions for various (difference, differential, integral, functional) quations.
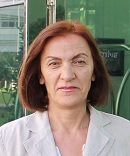
Prof. Ljiljana Petrovic, Department of Mathematics and Statistics, Faculty of Economics, University of Belgrade, SERBIA, e-mail: petrovl@ekof.bg.ac.rs
Title: "Some Generalization of Granger Causality and Stochastic Dynamic Systems"
Abstract: The basic idea in this paper is to relate some concepts of causality to the stochastic realization problem. More precisely, we consider the next problem (that follows directly from realization problem): how to find Markovian representations (even minimal) for a given family of Hilbert spaces (understood as outputs of a stochastic dynamic system S1 provided it is in a certain causality relationship with another family of Hilbert spaces (i.,e. with some informations about states of a stochastic dynamic system S2. Granger causality (C.W.J. Granger, Investigation Causal Relations by Econometric Models and Cross Spectral Methods, Econometrica. 37, 1969, 424-438) is one of the most popular measures to reveal causality influence of time series widely applied in economics, demography, neuroscience etc. The study of Granger-causality has been mainly preoccupied with time series. We shall instead concentrate on continuous time processes because many of systems to which it is natural to apply tests of causality, take place in continuous time. For example, this is generally the case within economy, finance, physics, medicine etc. First, we give various concepts of causality relationship between flows of information represented by families of Hilbert spaces. We then relate some concepts of causality to the stochastic realization problem. The approach adopted in this paper is that of [3]. However, since our results do not depend on probability distribution, we deal with arbitrary Hilbert spaces instead of those generated by Gaussian processes. Let us suppose that a stochastic dynamic system S1 causes, in a certain sense, behavior of some other stochastic dynamic system S2 . It is natural to assume that outputs H of system S1 can be registered and that some information E about states (or perhaps states themselves) of system S2 is given. Results we shall prove will tell us under which conditions concerning the relationships between H and E it is possible to find states\ G of system S1 which are in a certain causality relationship with H and E. It is clear that all given results can be extended to the case when a flows of informations are families of σ-algebras generated by finite dimensional Gaussian random variables. But, in the case that σ-algebras are arbitrary, not necessarily generated by Gaussian random variables the extensions of the proofs from this paper is nontrivial because one can not take an orthogonal complement with respect to a σ-algebra as one can with respect to subspace in Hilbert space.
Paper Submission
Papers must be written in the correct format: MS Word.doc or LaTeX or LaTeX2 and must be uploaded using the following form as .doc, or .pdf file. Invited and Regular Papers will be published in various Indexed Journals based on quality and reviewers' recommendations. Only full papers will be promoted to reviewers for thorough peer review. The deadline for paper submission expired. We do not accept papers any longer.
Organizing Committee
General Chairs (Editors)
-
Prof. Panos M. Pardalos,
Distinguished Prof. of Industrial and
Systems Engineering,
University of Florida, USA -
Professor Ravi P. Agarwal
Department of Mathematics
Texas A&M University - Kingsville
700 University Blvd.
Kingsville, TX 78363-8202, USA -
Prof. Ljubiša Kočinac, University of Nis,
Nis, Serbia
Senior Program Chair
- Prof. Valery Y. Glizer,
Ort Braude College, Karmiel, Israel
Program Chairs
-
Prof. Pierre Borne
ex IEEE France Section Chair, IEEE Fellow, IEEE/SMC Past President,
Ecole Centrale de Lille,
France -
Prof. Constantin Udriste,
University Politehnica of Bucharest,
Bucharest
Romania -
Prof. Marcia Cristina A. B. Federson,
Universidade de São Paulo,
São Paulo, Brazil
Tutorials Chair
-
Prof. Pradip Majumdar
Department of Mechanical Engineering
Northern Illinois University
Dekalb, Illinois, USA
Special Session Chair
-
Prof. Pavel Varacha
Tomas Bata University in Zlin
Faculty of Applied Informatics
Department of Informatics and Artificial Intelligence
Zlin, Czech Republic
Workshops Chair
-
Prof. Sehie Park,
The National Academy of Sciences,
Republic of Korea
Local Organizing Chair
-
Prof. Klimis Ntalianis,
Tech. Educ. Inst. of Athens (TEI), Athens, Greece
Publication Chair
-
Prof. Gen Qi Xu
Department of Mathematics
Tianjin University
Tianjin, China
Publicity Committee
- Prof. Vjacheslav Yurko,
Saratov State University,
Astrakhanskaya, Russia -
Prof. Myriam Lazard
Institut Superieur d' Ingenierie de la Conception
Saint Die, France
International Liaisons
- Professor Jinhu Lu, IEEE Fellow
Institute of Systems Science
Academy of Mathematics and Systems Science
Chinese Academy of Sciences
Beijing 100190, P. R. China - Prof. Olga Martin
Applied Sciences Faculty
Politehnica University of Bucharest
Romania - Prof. Vincenzo Niola
Departement of Mechanical Engineering for Energetics
University of Naples "Federico II"
Naples, Italy - Prof. Eduardo Mario Dias
Electrical Energy and Automation
Engineering Department
Escola Politecnica da Universidade de Sao Paulo
Brazil
Steering Committee
- Prof. Stefan Siegmund, Technische Universitaet Dresden, Germany
- Prof. Zoran Bojkovic, Univ. of Belgrade, Serbia
- Prof. Imre Rudas, Obuda University, Budapest, Hungary
Program Committee
A) Program Committee for PURE MATHEMATICS
- Prof. Ferhan M. Atici, Western KentuckyUniversity, Bowling Green, KY 42101, USA
- Prof. Ravi P. Agarwal, Texas A&M University - Kingsville, Kingsville, TX, USA
- Prof. Martin Bohner, Missouri University of Science and Technology, Rolla, Missouri, USA
- Prof. Dashan Fan, University of Wisconsin-Milwaukee, Milwaukee, WI, USA
- Prof. Paolo Marcellini. University of Firenze, Firenze, Italy
- Prof. Xiaodong Yan, University of Connecticut, Connecticut, USA
- Prof. Ming Mei, McGill University, Montreal, Quebec, Canada
- Prof. Enrique Llorens, University of Valencia, Valencia, Spain
- Prof. Yuriy V. Rogovchenko, University of Agder, Kristiansand, Norway
- Prof. Yong Hong Wu, Curtin University of Technology, Perth, WA, Australia
- Prof. Angelo Favini, University of Bologna, Bologna, Italy
- Prof. Andrew Pickering, Universidad Rey Juan Carlos, Mostoles, Madrid, Spain
- Prof. Guozhen Lu, Wayne state university, Detroit, MI 48202, USA
- Prof. Gerd Teschke, Hochschule Neubrandenburg - University of Applied Sciences, Germany
- Prof. Juan Carlos Cortes Lopez, Universidad Politecnica de Valencia, Spain
- Prof. Julian Lopez-Gomez, Universitad Complutense de Madrid, Madrid, Spain
- Prof. Jozef Banas, Rzeszow University of Technology, Rzeszow, Poland
- Prof. Kevin R. Payne, Universita' degli Studi di Milano, Milan, Italy
- Prof. Juan Pablo Rincon-Zapatero, Universidad Carlos III De Madrid, Madrid, Spain
- Prof. Valery Y. Glizer, ORT Braude College, Karmiel, Israel
- Prof. Norio Yoshida, University of Toyama, Toyama, Japan
- Prof. Feliz Minhos, Universidade de Evora, Evora, Portugal
- Prof. Mihai Mihailescu, University of Craiova, Craiova, Romania
- Prof. Lucas Jodar, Universitat Politecnica de Valencia, Valencia, Spain
- Prof. Dumitru Baleanu, Cankaya University, Ankara, Turkey
- Prof. Jianming Zhan, Hubei University for Nationalities, Enshi, Hubei Province, China
- Prof. Zhenya Yan, Institute of Systems Science, AMSS, Chinese Academy of Sciences, Beijing, China
- Prof. Nasser-Eddine Mohamed Ali Tatar, King Fahd University of Petroleum and Mineral, Dhahran, Saudi Arabia
- Prof. Jianqing Chen, Fujian Normal University, Cangshan, Fuzhou, Fujian, China
- Prof. Josef Diblik, Brno University of Technology, Brno, Czech Republic
- Prof. Stanislaw Migorski, Jagiellonian University in Krakow, Krakow, Poland
- Prof. Qing-Wen Wang, Shanghai University, Shanghai, China
- Prof. Luis Castro, University of Aveiro, Aveiro, Portugal
- Prof. Alberto Fiorenza, Universita' di Napoli "Federico II", Napoli (Naples), Italy
- Prof. Patricia J. Y. Wong, Nanyang Technological University, Singapore
- Prof. Salvatore A. Marano, Universita degli Studi di Catania, Catania, Italy
- Prof. Sung Guen Kim, Kyungpook National University, Daegu, South Korea
- Prof. Maria Alessandra Ragusa, Universita di Catania, Catania, Italy
- Prof. Gerassimos Barbatis, University of Athens, Athens, Greece
- Prof. Jinde Cao, Distinguished Prof., Southeast University, Nanjing 210096, China
- Prof. Kailash C. Patidar, University of the Western Cape, 7535 Bellville, South Africa
- Prof. Mitsuharu Otani, Waseda University, Japan
- Prof. Luigi Rodino, University of Torino, Torino, Italy
- Prof. Carlos Lizama, Universidad de Santiago de Chile, Santiago, Chile
- Prof. Jinhu Lu, Chinese Academy of Sciences, Beijing, China
- Prof. Narcisa C. Apreutesei, Technical University of Iasi, Iasi, Romania
- Prof. Sining Zheng, Dalian University of Technology, Dalian, China
- Prof. Daoyi Xu, Sichuan University, Chengdu, China
- Prof. Zili Wu, Xi'an Jiaotong-Liverpool University, Suzhou, Jiangsu, China
- Prof. Wei-Shih Du, National Kaohsiung Normal University, Kaohsiung City, Taiwan
- Prof. Khalil Ezzinbi, Universite Cadi Ayyad, Marrakesh, Morocco
- Prof. Youyu Wang, Tianjin University of Finance and Economics, Tianjin, China
- Prof. Satit Saejung, Khon Kaen University, Thailand
- Prof. Chun-Gang Zhu, Dalian University of Technology, Dalian, China
- Prof. Mohamed Kamal Aouf, Mansoura University, Mansoura City, Egypt
- Prof. Yansheng Liu, Shandong Normal University, Jinan, Shandong, China
- Prof. Naseer Shahzad, King Abdulaziz University, Jeddah, Saudi Arabia
- Prof. Janusz Brzdek, Pedagogical University of Cracow, Poland
- Prof. Mohammad T. Darvishi, Razi University, Kermanshah, Iran
- Prof. Ahmed El-Sayed, Alexandria University, Alexandria, Egypt
B) Program Committee for APPLIED MATHEMATICS AND COMPUTATIONAL METHODS - Prof. Martin Bohner, Missouri University of Science and Technology, Rolla, Missouri, USA
- Prof. Martin Schechter, University of California, Irvine, USA
- Prof. Xiaodong Yan, University of Connecticut, Connecticut USA
- Prof. Ravi P. Agarwal, Texas A&M University - Kingsville, Kingsville, TX, USA
- Prof. Yushun Wang, Nanjing Normal university, Nanjing, China
- Prof. Patricia J. Y. Wong, Nanyang Technological University, Singapore
- Prof. Andrei Korobeinikov, Centre de Recerca Matematica, Barcelona, Spain
- Prof. Jim Zhu, Western Michigan University, Kalamazoo, MI, USA
- Prof. Ferhan M. Atici, Department of Mathematics, Western Kentucky University, USA
- Prof. Gerd Teschke, Institute for Computational Mathematics in Science and Technology, Neubrandenburg, Berlin-Dahlem, Germany
- Prof. Meirong Zhang, Tsinghua University, Beijing, China
- Prof. Lucio Boccardo, Universita degli Studi di Roma "La Sapienza", Roma, Italy
- Prof. Shanhe Wu, Longyan University, Longyan, Fujian, China
- Prof. Natig M. Atakishiyev, National Autonomous University of Mexico, Mexico
- Prof. Jianming Zhan, Hubei University for Nationalities, Enshi, Hubei Province, China
- Prof. Narcisa C. Apreutesei, Technical University of Iasi, Iasi, Romania
- Prof. Chun-Gang Zhu, Dalian University of Technology, Dalian, China
- Prof. Abdelghani Bellouquid, University Cadi Ayyad, Morocco
- Prof. Jinde Cao, Southeast University/ King Abdulaziz University, China
- Prof. Josef Diblik, Brno University of Technology, Brno, Czech Republic
- Prof. Jianqing Chen, Fujian Normal University, Fuzhou, Fujian, China
- Prof. Naseer Shahzad, King Abdulaziz University, Jeddah, Saudi Arabia
- Prof. Sining Zheng, Dalian University of Technology, Dalian, China
- Prof. Leszek Gasinski, Uniwersytet Jagielloński, Krakowie, Poland
- Prof. Satit Saejung, Khon Kaen University, Muang District, Khon Kaen, Thailand
- Prof. Juan J. Trujillo, Universidad de La Laguna, La Laguna, Tenerife, Spain
- Prof. Tiecheng Xia, Department of Mathematics, Shanghai University, China
- Prof. Stevo Stevic, Mathematical Institute Serbian Academy of Sciences and Arts, Beogrand, Serbia
- Prof. Lucas Jodar, Universitat Politecnica de Valencia, Valencia, Spain
- Prof. Noemi Wolanski, Universidad de Buenos Aires, Buenos Aires, Argentina
- Prof. Zhenya Yan, Chinese Academy of Sciences, Beijing, China
- Prof. Juan Carlos Cortes Lopez, Universidad Politecnica de Valencia, Spain
- Prof. Wei-Shih Du, National Kaohsiung Normal University, Kaohsiung City, Taiwan
- Prof. Kailash C. Patidar, University of the Western Cape, Cape Town, South Africa
- Prof. Hossein Jafari, University of Mazandaran, Babolsar, Iran
- Prof. Abdel-Maksoud A Soliman, Suez Canal University, Egypt
- Prof. Janusz Brzdek, Pedagogical University of Cracow, Cracow, Poland
- Dr. Fasma Diele, Italian National Research Council (C.N.R.), Bari, Italy.
Topics of Interest
PURE MATHEMATICS: Analysis, Nonlinear Analysis, Algebra, Topology, Algebraic Topology, Lie Groups and Lie Algebras, C* Algebras, Functional Analysis, Geometry, Differential Geometry, Algebraic Geometry, Complex Analysis, Set Theory, Operators' Theory, Ordinary Differential Equations And Chaos, Partial Differential Equations, Inverse Problems, Banach Spaces, Convex Analysis, Ergodic Theory, Ergodic Theory and Symbolic Dynamics, Graph Theory, Manifolds, Modern Group Theory, Rings & Modules & Representations, Fields and Galois Theory, Algebraic K-Theory, Commutative Algebra, Harmonic Analysis, Measure Theory, Dynamical Systems, Topological Algebras, Mathematical Logic, Algebraic Number Theory, Analytic Number Theory, Probability & Statistics, Combinatorics, Combinatorics and Discrete Geometry, Module and Representation Theory, Non-Smooth Analysis, Mathematical Physics, Theoretical Topics in Numerical Analysis, Optimization and Variational Analysis, Theoretical Game Theory, Theoretical Fluid Dynamics, Theoretical Foundations of Control, Algorithms and Complexity, Others
APPLIED MATHEMATICS AND COMPUTATIONAL METHODS: Mathematical Logic, Probability, Statistics, Combinatorics, Discrete Mathematics, Numerical Analysis, Computational sciences, Applied Functional Analysis, Differential Geometry, Systems Theory, Computational Geometry, Control Theory, Numerical ODEs, Numerical PDEs, Scientific Computing and Algorithms, Stochastic Differential Equations, Approximation Theory, Numerical Linear Algebra, Numerical Integral Equations, Error Analysis and Interval Analysis, Difference Equations and Recurrence Relations, Algorithms & Computational Complexity, Computational Intelligence, Artificial Intelligence, Dynamical Systems, Computational Physics, Computational Statistics, Computational Chemistry, Computational Engineering, Mathematical Biology, Mathematical Medicine, Mathematics in Fluid mechanics, Mathematics in Heat and Mass Transfer, Theoretical Mechanics, Optimization and Operational Research, Others