* Invited and Regular Papers will be published in various Indexed Journals (ISI, SCOPUS, EBSCO, DBLP, ACM, IET/INSPEC, Proquest, Copernicus etc...) based on quality and reviewers' recommendations or in our Books in Springer Verlag. Click here
* Authors will be informed about the title of the Journal before
their registration.
* Contact us by email: support@inase.org Registration fees Publication Ethics and Malpractice Statement Deadlines
PLENARY SPEAKERS:
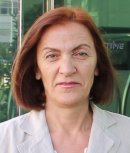
Prof. Ljiljana Petrovic, Department of Mathematics and Statistics, Faculty of Economics, University of Belgrade, SERBIA, e-mail: petrovl@ekof.bg.ac.rs
Title: "Statistical Causality Between Flows of Information"
Abstract: Causality is a topic which nowadays receives much attention. Many scientist in statistics, computer science (especially those in artificial intelligence), physics, econometrics, medicine and philosophy are investigating questions like "what would have happened if" and "what would happen if". Causality is, in any case, a prediction property and the central question is: is it possible to reduce available information in order to predict a given filtration?
Granger causality (C.W.J. Granger, Investigation Causal Relations by Econometric Models and Cross Spectral Methods, Econometrica. 37, 1969, 424-438) is one of the most popular measures to reveal causality influence of time series widely applied in economics, demography, neuroscience etc. The study of Granger-causality has been mainly preoccupied with time series. We shall instead concentrate on continuous time processes because many of systems to which it is natural to apply tests of causality, take place in continuous time. For example, this is generally the case within economy, finance, physics, medicine etc. First, we give various concepts of causality relationship between flows of information represented by families of Hilbert spaces and flows of information represented by filtrations. Then, we extend the given causality concept from fixed times to stopping times, i.e. we give a characterization of causality using σ-field associated to stopping times. The given definitions can be applied to stochastic processes.
Then, we apply the given concepts of causality on weak solutions of stochastic differential equations (SDE) with driving semimartingales. More precisely, we show equivalence between the given models of causality and weak uniqueness of regular solutions of SDE with driving semimartingales.
The causality concept is closely connected to extremality of martingale problem. Also, the statistical causality is very closely linked to the concept of extremality of measures and to the concept of adapted distribution.
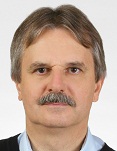
Prof. Janusz Brzdek, Department of Mathematics of Pedagogical University of Cracow, POLAND, e-mail: jbrzdek@up.krakow.pl
Title: "Connections Between Ulam Stability and Fixed Points Theory"
Abstract: The issue of Ulam stability of an equation can be very roughly expressed in the following way: When a function satisfying an equation approximately (in some sense) must be close to an exact solution to the equation? It has been motivated by a problem formulated by S.M. Ulam in 1940 and an answer to it published in 1941 by D.H. Hyers. At present, it is a large field of investigations and concerns various types of equations (e.g., difference, differential, functional, integral).
Numerous results on Ulam stability can be stated in the form of fixed point theorems in some function spaces (for various operators, also nonlinear) and some fixed point theorems can be applied to prove stability for equations of different kinds; moreover, some new fixed point results have been obtained in recent years in connection with investigations of Ulam's stability. We discuss those connections, provide several recent particular examples of them and show some methods of proofs of various stability outcomes. We also present some basic definitions and examples of simple classical results.
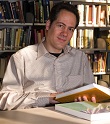
Prof. Ansgar Steland, RWTH Aachen University, GERMANY, e-mail: steland@stochastik.rwth-aachen.de
Title: "New Large Sample Approximations for Covariance Matrices in High Dimensions"
Abstract: The inferential analysis of the correlation structure of high dimensional vector time series plays an important role in data science and big data. The issue arises when observing a large number d of time series where d may be larger than the sample size n. A common approach is to rely on regularized estimators, for which, however, there is a lack of asymptotic theory which would allow us to construct valid inferential procedures such as hypothesis tests or confidence intervals. We discuss recent results which provide appropriate large sample asymptotics, in the sense of strong or weak approximations by Wiener processes, which allow us to base statistical inference on projections of the high-dimensional data onto subspaces spanned by l1-sparse vectors. Such l1-sparse vectors arise naturally in several applications such as portfolio optimization or sparse principal component analysis, which are discussed in some detail. As a further interesting application we discuss shrinkage estimators of covariance matrices, which shrink the sample variance-covariance matrix towards a simple target resulting in a regular estimator with improved performance. Known limit theorems dealing with high dimensional data usually work under some constraint on the the dimension d and the sample size n, as in classical random matrix theory. Contrary, the results presented here impose no constraints on d.
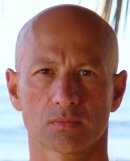
Prof. Nodari Vakhania, Centro de Investigacion en Ciencias, UAEMor, MEXICO, e-mail: nodari@uaem.mx
Title: "Some Complexity Classes of the Combinatorial Optimization Problems"
Abstract: In this talk we discuss about complexity classes of the combinatorial optimization problems. Interestingly, two very similar such problems may have a drastically different computational complexity. Just by modifying one parameter of a given NP-hard com- binatorial optimization problem may turn it to a polynomially solvable one. We shall give some examples of such problems and will discuss the mathematical background for such an unexpected mutation of the complexity status. Below we give a short survey of the related concepts.
Combinatorial optimization problems constitute a significant class of practical problems with a discrete nature. They have emerged in late 40-s of 20th century. With a rapid grow of the industry, the new demands in the optimal solution of the newly emerged resource management and distribution problems have arisen. For the development of effective solution methods, these problems were formalized and addressed mathematically.
The combinatorial optimization problems are partitioned into two basic types, type P, which are polynomially solvable ones, and intractable NP-hard problems. It is be- lieved that it's very unlikely that an NP-hard problem can be solved in polynomial time.
In this talk we consider some scheduling problems which constitute an important class of the discrete optimization problems. They deal with a finite set of requests called jobs to be performed (or scheduled) on a finite (and limited) set of resources called machines (or processors). The aim is to choose the order of processing the jobs on machines so as to meet a given objective criteria.